Introduction to Digital Twin Technology
The concept of a Digital Twin has been gaining traction in recent years as a powerful tool for optimizing manufacturing processes, improving product design, and enhancing overall operational efficiency. A Digital Twin is a virtual representation of a physical object, process, or system that accurately reflects its real-world counterpart throughout its lifecycle. This technology leverages data from sensors, simulations, and historical information to create a dynamic, real-time model that can be used for various purposes, such as monitoring, analysis, and prediction.
In the context of manufacturing, the Digital Twin is often referred to as Virtual Manufacturing. It involves creating a digital replica of the entire manufacturing process, from product design to production, and even extending to the end-of-life stage. By utilizing a Digital Twin, manufacturers can gain valuable insights into their processes, identify potential issues, and make data-driven decisions to optimize their operations.
Key Components of a Digital Twin
To create an effective Digital Twin, several key components must be in place:
-
Physical Asset: The physical object, process, or system that the Digital Twin represents. This could be a single machine, an entire production line, or even a complete factory.
-
Sensors and Data Collection: Sensors are used to gather real-time data from the physical asset, such as temperature, pressure, vibration, and performance metrics. This data is continuously fed into the Digital Twin to ensure its accuracy and relevance.
-
Data Integration and Processing: The collected data is integrated and processed using various techniques, such as data fusion, machine learning, and artificial intelligence. This enables the Digital Twin to provide meaningful insights and predictions.
-
Simulation and Modeling: The Digital Twin incorporates simulation and modeling capabilities to analyze the behavior of the physical asset under different conditions. This allows for the exploration of “what-if” scenarios and the optimization of processes.
-
Visualization and User Interface: A user-friendly interface is essential for interacting with the Digital Twin, visualizing data, and making informed decisions. This may include dashboards, 3D models, and virtual reality or augmented reality experiences.
Benefits of Implementing a Digital Twin in Manufacturing
Implementing a Digital Twin in manufacturing offers numerous benefits, including:
1. Improved Product Design and Development
By creating a virtual replica of a product, designers and engineers can test and refine their designs without the need for physical prototypes. This accelerates the product development process, reduces costs, and enables the exploration of multiple design iterations. The Digital Twin can simulate various conditions, such as stress, temperature, and wear, to predict product performance and identify potential issues early in the development cycle.
2. Enhanced Process Optimization
A Digital Twin of the manufacturing process allows for real-time monitoring and analysis of production lines. By collecting data from sensors and machines, the Digital Twin can identify bottlenecks, inefficiencies, and potential equipment failures. This information can be used to optimize production schedules, reduce downtime, and improve overall equipment effectiveness (OEE). The Digital Twin can also simulate process changes and predict their impact, enabling manufacturers to make informed decisions and continuously improve their operations.
3. Predictive Maintenance and Asset Management
One of the most significant benefits of a Digital Twin is its ability to enable predictive maintenance. By analyzing real-time data and historical trends, the Digital Twin can predict when a machine or component is likely to fail, allowing for proactive maintenance interventions. This minimizes unplanned downtime, extends asset life, and reduces maintenance costs. The Digital Twin can also optimize maintenance schedules based on actual asset condition, rather than relying on fixed intervals, further improving efficiency and cost-effectiveness.
4. Supply Chain Optimization
A Digital Twin can be extended beyond the boundaries of a single factory to encompass the entire supply chain. By creating a virtual representation of the supply chain, manufacturers can gain visibility into the flow of materials, identify potential disruptions, and optimize logistics. The Digital Twin can simulate various scenarios, such as changes in demand, supplier performance, or transportation routes, to help manufacturers make informed decisions and adapt to changing conditions.
5. Training and Skill Development
Digital Twins can be used to create immersive training experiences for operators and maintenance personnel. By leveraging virtual reality or augmented reality technologies, employees can be trained on complex tasks or procedures in a safe, controlled environment. This reduces the risk of accidents, improves training effectiveness, and allows for the development of skills without disrupting production. The Digital Twin can also be used to capture and preserve the knowledge of experienced workers, ensuring that their expertise is not lost when they retire or leave the organization.
Challenges and Considerations in Implementing a Digital Twin
While the benefits of a Digital Twin are significant, implementing this technology also presents some challenges and considerations:
1. Data Quality and Integration
Creating an accurate and reliable Digital Twin requires high-quality data from various sources, such as sensors, machines, and enterprise systems. Ensuring data accuracy, consistency, and timely integration can be challenging, especially in legacy manufacturing environments with disparate systems and protocols. Manufacturers need to invest in robust data collection, processing, and integration infrastructure to support their Digital Twin initiatives.
2. Cybersecurity and Data Privacy
As Digital Twins rely on real-time data from connected devices and systems, cybersecurity becomes a critical concern. Manufacturers must implement strong security measures to protect their data and systems from unauthorized access, tampering, or theft. This includes implementing secure Communication Protocols, access controls, and data encryption. Additionally, manufacturers must ensure compliance with relevant data privacy regulations, such as GDPR or CCPA, when collecting and processing data for their Digital Twins.
3. Skill and Expertise Requirements
Implementing and maintaining a Digital Twin requires a diverse set of skills and expertise, including data science, software development, domain knowledge, and IT infrastructure. Manufacturers may need to invest in training their existing workforce or hire new talent with the necessary skills. Collaboration between IT, OT (Operational Technology), and domain experts is crucial for the successful implementation and operation of a Digital Twin.
4. Integration with Existing Systems and Processes
Integrating a Digital Twin into existing manufacturing systems and processes can be complex and time-consuming. Manufacturers need to ensure that their Digital Twin seamlessly integrates with their Enterprise Resource Planning (ERP), Manufacturing Execution Systems (MES), and other relevant systems. This may require adapting existing processes, workflows, and interfaces to accommodate the Digital Twin. A phased approach to implementation can help manage complexity and risk.
5. Scalability and Performance
As the complexity and scope of a Digital Twin grow, scalability and performance become critical considerations. The Digital Twin must be able to handle large volumes of data, perform complex simulations, and provide real-time insights without compromising performance. Manufacturers need to design their Digital Twin architecture with scalability in mind, leveraging cloud computing, edge processing, and other technologies to ensure optimal performance.
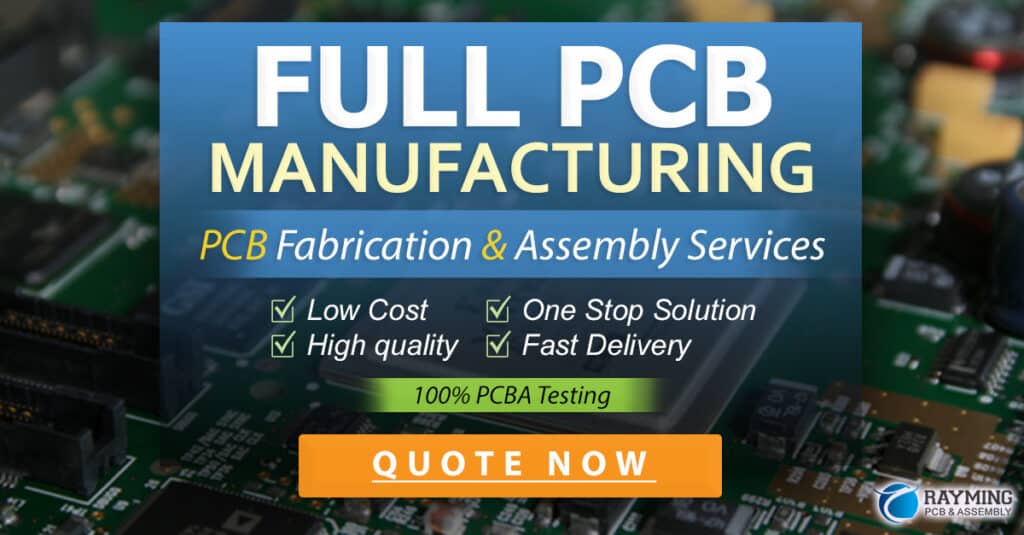
Case Studies and Real-World Applications
To illustrate the potential of Digital Twins in manufacturing, let’s explore some real-world case studies:
1. General Electric (GE) – Digital Wind Farm
GE has implemented a Digital Twin for its wind turbines, creating a virtual replica of each turbine in a wind farm. The Digital Twin collects data from sensors on the turbines, weather data, and historical performance data to optimize the operation and maintenance of the wind farm. By analyzing this data, GE can predict when a turbine is likely to fail, schedule proactive maintenance, and optimize the placement of new turbines to maximize energy production. The Digital Wind Farm has resulted in increased energy output, reduced downtime, and lower maintenance costs for GE and its customers.
2. Siemens – Digital Factory
Siemens has developed a comprehensive Digital Twin solution for its manufacturing operations, known as the Digital Factory. The Digital Factory creates a virtual representation of the entire production process, from product design to final assembly. By simulating various production scenarios, Siemens can optimize its production lines, reduce cycle times, and improve product quality. The Digital Factory also enables predictive maintenance, reducing unplanned downtime and extending the life of production assets. Siemens has reported significant cost savings, increased productivity, and faster time-to-market as a result of its Digital Twin implementation.
3. Boeing – Digital Twin for Aircraft Design and Maintenance
Boeing has embraced Digital Twin technology to optimize the design, production, and maintenance of its aircraft. By creating a virtual replica of an aircraft, Boeing can simulate various flight conditions, test design changes, and predict maintenance requirements. The Digital Twin also enables Boeing to monitor the performance of its aircraft in real-time, collecting data from sensors and onboard systems. This data is used to optimize flight routes, reduce fuel consumption, and schedule predictive maintenance. Boeing’s Digital Twin initiative has resulted in improved aircraft performance, reduced maintenance costs, and enhanced passenger safety.
Future Trends and Developments in Digital Twin Technology
As Digital Twin technology continues to evolve, several trends and developments are shaping its future in manufacturing:
1. Integration with AI and Machine Learning
The integration of Artificial Intelligence (AI) and Machine Learning (ML) with Digital Twins is expected to unlock new possibilities for process optimization and predictive analytics. AI and ML algorithms can analyze the vast amounts of data generated by Digital Twins, identifying patterns, anomalies, and optimization opportunities. This will enable manufacturers to make more accurate predictions, automate decision-making, and continuously improve their processes.
2. Expansion to Product Lifecycle Management
Digital Twins are expected to play a larger role in Product Lifecycle Management (PLM), extending beyond the manufacturing phase to encompass the entire product lifecycle. This includes using Digital Twins for product design, simulation, testing, and even post-sale monitoring and support. By creating a comprehensive Digital Twin of a product, manufacturers can gain valuable insights into product performance, customer usage patterns, and potential improvements for future iterations.
3. Collaborative and Networked Digital Twins
As Digital Twin technology matures, there is a growing trend towards collaborative and networked Digital Twins. This involves creating a network of interconnected Digital Twins that can share data, insights, and best practices across different manufacturing sites, suppliers, and even industry partners. Collaborative Digital Twins can foster innovation, optimize supply chains, and create new business models based on shared data and expertise.
4. Integration with Edge Computing and 5G
The proliferation of edge computing and 5G networks is expected to enhance the capabilities of Digital Twins in manufacturing. Edge computing allows for real-time data processing and analysis close to the data source, reducing latency and enabling faster decision-making. 5G networks provide high-speed, low-latency connectivity, enabling the seamless integration of sensors, machines, and Digital Twins. This combination of edge computing and 5G will support the deployment of more sophisticated and responsive Digital Twins in manufacturing environments.
5. Augmented and Virtual Reality Applications
Augmented Reality (AR) and Virtual Reality (VR) technologies are expected to play a significant role in the future of Digital Twins. AR and VR can provide immersive experiences for interacting with Digital Twins, enabling users to visualize, manipulate, and analyze virtual representations of products and processes. This can enhance collaboration, training, and decision-making, allowing manufacturers to leverage the full potential of their Digital Twins.
Frequently Asked Questions (FAQ)
1. What is the difference between a Digital Twin and a simulation?
A Digital Twin is a virtual representation of a physical object, process, or system that is continuously updated with real-time data from its physical counterpart. It combines simulation, data analytics, and machine learning to provide a comprehensive, dynamic view of the asset. In contrast, a simulation is a static model that represents a system at a particular point in time, based on predefined parameters and assumptions. While simulations are useful for analyzing specific scenarios, Digital Twins offer a more dynamic and holistic approach to understanding and optimizing assets throughout their lifecycle.
2. How does a Digital Twin handle data privacy and security?
Data privacy and security are critical considerations in the implementation of Digital Twins. Manufacturers must ensure that they have robust security measures in place to protect the data collected and processed by their Digital Twins. This includes implementing secure communication protocols, access controls, and data encryption to prevent unauthorized access or tampering. Additionally, manufacturers must comply with relevant data privacy regulations, such as GDPR or CCPA, when collecting and processing personal data. Regular security audits, employee training, and the adoption of best practices in data governance can help mitigate privacy and security risks associated with Digital Twins.
3. What are the key steps in implementing a Digital Twin in manufacturing?
Implementing a Digital Twin in manufacturing typically involves the following key steps:
- Define the scope and objectives of the Digital Twin project, identifying the assets, processes, or systems to be virtualized.
- Assess the existing data infrastructure, identifying data sources, quality, and integration requirements.
- Develop the data collection and processing infrastructure, installing sensors, and establishing data pipelines.
- Create the Digital Twin model, incorporating simulation, analytics, and visualization capabilities.
- Integrate the Digital Twin with existing systems, such as ERP, MES, and PLM.
- Test and validate the Digital Twin, ensuring accuracy, performance, and usability.
- Deploy the Digital Twin in the production environment, providing training and support to end-users.
- Continuously monitor, update, and optimize the Digital Twin based on real-time data and user feedback.
4. How can a Digital Twin help with product design and development?
A Digital Twin can significantly enhance product design and development by allowing designers and engineers to create virtual prototypes of products, test them under various conditions, and refine their designs without the need for physical prototypes. This accelerates the development process, reduces costs, and enables the exploration of multiple design iterations. The Digital Twin can simulate various factors, such as stress, temperature, and wear, to predict product performance and identify potential issues early in the development cycle. This helps manufacturers create more reliable, efficient, and customer-centric products, while reducing time-to-market and development costs.
5. What are the potential challenges in scaling a Digital Twin across multiple manufacturing sites?
Scaling a Digital Twin across multiple manufacturing sites can present several challenges, including:
- Data integration and standardization: Ensuring that data from different sites is consistent, accurate, and easily accessible can be complex, especially if the sites use different systems and protocols.
- Infrastructure compatibility: The hardware and software infrastructure at each site may vary, requiring adaptations or upgrades to support the Digital Twin.
- Skill and resource availability: Implementing and maintaining Digital Twins requires specialized skills and resources, which may not be equally available at all sites.
- Governance and coordination: Managing a Digital Twin across multiple sites requires clear governance structures, roles, and responsibilities to ensure consistent implementation and operation.
- Security and compliance: Scaling a Digital Twin across sites may introduce new security and compliance risks, requiring a robust, multi-site security strategy and governance framework.
To address these challenges, manufacturers should develop a clear roadmap for scaling their Digital Twin, invest in data and infrastructure standardization, provide training and support to local teams, and establish strong governance and coordination mechanisms.
Conclusion
Digital Twin technology, or Virtual Manufacturing, is a powerful tool for optimizing manufacturing processes, improving product design, and enhancing operational efficiency. By creating a virtual representation of physical assets, processes, or systems, manufacturers can gain valuable insights, predict performance, and make data-driven decisions. The benefits of implementing a Digital Twin in manufacturing are numerous, including improved product design and development, enhanced process optimization, predictive maintenance, supply chain optimization, and improved training and skill development.
However, implementing a Digital Twin also presents challenges, such as ensuring data quality and integration, addressing cybersecurity and data privacy concerns, acquiring the necessary skills and expertise, integrating with existing systems and processes, and ensuring scalability and performance. To overcome these challenges, manufacturers must invest in robust data infrastructure, foster collaboration between IT, OT, and domain experts, and adopt a phased approach to implementation.
Real-world case studies from companies like General Electric, Siemens, and Boeing demonstrate the tangible benefits of Digital Twin technology in manufacturing, from increased energy output and reduced maintenance costs to improved product quality and faster time-to-market. As Digital Twin technology continues to evolve, the integration of AI and machine learning, expansion to product lifecycle management, the emergence of collaborative and networked Digital Twins, integration with edge computing and 5G, and the adoption of augmented and virtual reality applications will shape the future of Virtual Manufacturing.
By embracing Digital Twin technology, manufacturers can unlock new levels of efficiency, agility, and innovation, positioning themselves for success in the increasingly competitive and dynamic manufacturing landscape.
No responses yet